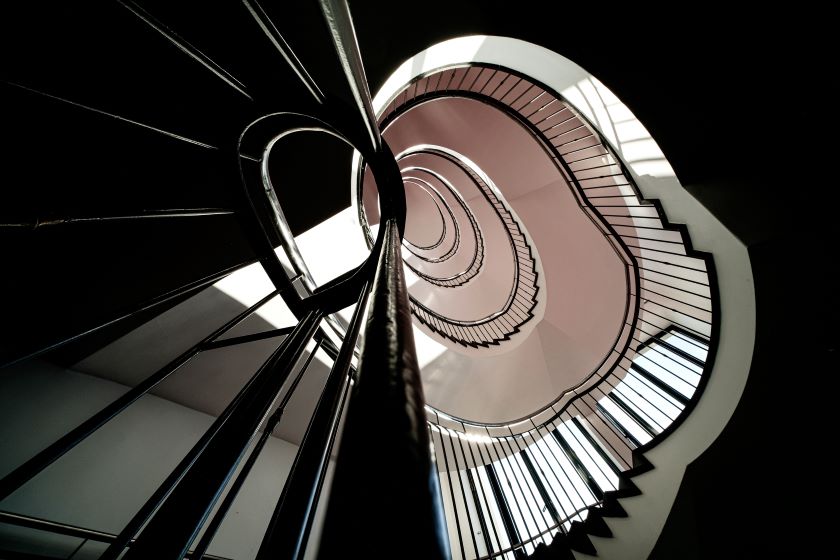
© Joachim Löning
Abstract
Our solutions provide parameters thru regression analysis and other methods. For end customers such methods are too complex to understand in a short time period. Investment advisors are in a perfect position to deliver suitable narratives for every customer. Such bridging of the gap of explainability is more difficult for robo-advisors. The article describes a growing momentum for explainable artificial intelligence (XAI) and summarizes how our tools are giving helpful hints for advisors to deliver narratives of investment advice.
Dealing with complexity is a constant challenge in investment consulting. Advisors who manage to convey the state of affairs to their clients with ease deliver immediate value. The client is spared the time-consuming task of dealing with an important topic that is perceived as complicated.
The meaningful narrative, the advisor offers, is necessarily limited to snippets of reality while establishing the client’s trust in the advisor’s competence. For the customer, it becomes tangible why the consultant is better than a cheaper do-it-yourself method. Narratives strengthen the client’s confidence in delegating his investment decisions and should be actively sought by the advisor.
This is not about the question of which methods the “right” investment advisor uses, but rather about providing competent advice. This can be broken down into the two components: specialist knowledge and advisory competence. A good advisor should be able to use both elements flexibly. As a rule, he or she has software-supported tools at his or her disposal to handle the specialist part. The advisor’s competence becomes tangible as a narrative, with which the consultant builds a bridge from the calculated software-supported parameters to the insight of the client.
Software-based algorithms for calculating the individual parameters of investment advice are also available from a Robo-Advisor. However, the advantage of the flexibility of a human counterpart, who can respond to his client individually, is missing there. With a Robo-Advisor’s offer, this flexibility is not available. This is due to the business model, because the point is to do without human support. The flexibility of a human advisor is exactly what a robot cannot master.
Crucial to the usefulness of software-supported narratives in advisory (and required by law) is the explainability of the methods used. This is how the European General Data Protection Regulation (GDPR), which has been in force since 2018, defines the duty to explain. The customer has a right to transparency in data collection and processing. Whether a customer’s investment is governed by a contract is subject to the requirements of the GDPR on profiling is not discussed here. What is clear, however, is the demand for complete transparency in the question of why a customer has been classified as based on his personal data.
Very specifically dedicated to explainability is a technical term that can be translated as “explainable artificial intelligence” and is common under eXplainable Artificial Intelligence or XAI. The main aim here is to make the results of mechanical processes understandable and comprehensible. It is people who bear responsibility and people who should not be blindly subjected to the result of an automatic algorithm. While XAI was initially concerned with leaving the decision to drone operators, the analogy with the role of the advisor on the investment of money cannot be dismissed. The consultant should be able to understand how to dress machine-generated results into a narrative for the client.
Gunnell (2017), for example, demands that results obtained with methods of artificial intelligence should be understandable. This does not mean that the consultant must comprehensively penetrate the algorithms used – it is sufficient to understand why a certain result is not achieved, to know when something is right or wrong, when a machine result can be trusted and when an error has probably occurred. This is important when it comes to making a far-reaching decision using methods of artificial intelligence. Humans should have the “last word”, must take responsibility for it and therefore understand the results of powerful algorithms. Algorithms calculate the basis for far-reaching decisions on the financial future of the client. the client will hold the advisor responsible and the advisor must therefore understand what is happening.
With a look at the standard procedure of Modern Portfolio Theory, the challenge of explaining investment may become clear. There, regression models are used whose behaviour is difficult for the user to understand. To avoid cluster risks and thus to optimize diversification in a portfolio, the variance/covariance matrix, also known as the Markowitz method, is the method of choice. The correlations used describe the behaviour of securities in relation to each other and can only be intuitively understood by very few people. As a result of an optimization calculation, recommendations are sold and bought. The answer why this is done is simple (better diversification effect in relation to the other values in the portfolio) but also complex, because even minor modifications to the initial depot change the result and many customers and consultants find such system behaviour complicated and unsatisfactory.
Not only the methods and algorithms for investing money are complex, but also established products and product categories for investing money are increasingly being questioned. Mittnik (2020), who has taken a look at the extraordinarily complex construction rules of indices and thus passive funds, provides interesting reading on the topic of complexity in passive and active funds.
The examples show why it is important for an advisor to offer his clients intuitively understandable narratives. The advisor does not need to fully understand the complexity of algorithmically generated parameters or the complex details behind the most recently successful indices, but if he has the right mix of expertise and advisory skills, as formulated at the beginning, he will explain the meaning to his client.
We rise to the challenge of explainability. The consultant should not be afraid to explain to the client that regression analytical methods come from the toolbox of artificial intelligence and are “naturally” difficult to explain. We started early to visualize the Markowitz Optimization, made the portfolio tangible with touch gestures and in a completely new tool we offer the consultant interpretation of the results of the portfolio analysis. Between the visualization of portfolio content and the (AI) methods running in the background, we have built the so-called Quality Box as a connecting element. In this box, the consultant is offered explanatory aids which he translates into individual narratives for the client. This interpretation layer is itself generated with machine processes, but the methods used there are rule-based and can therefore be explained clearly and intuitively.
This is precisely how the advisor can differentiate himself from a Robo-Advisor offer and strengthen the client’s confidence in delegating the investment decision.
Literature:
Prof. Stefan Mittnik: Kostolanys Depot, FAS v. 2.8.20, Seite 28
David Gunning: DARPA/I2O Program Update November 2017, PDF Document